Estimating commercial fish production in Santa Catarina, Brazil, based on animal transport records and mathematical models
DOI:
https://doi.org/10.52945/rac.v38i1.1895Keywords:
Fish aquaculture, Forecast, Tilapia, TroutAbstract
This study investigated the correlation between fish transit data, recorded in the Animal Transit Guides (GTA), and fish farming production estimates to develop mathematical models to predict commercial fish production in Santa Catarina. GTA data provided by Cidasc and production estimates made by Epagri between 2015 and 2021 were used. The data was combined and linear regression models were developed based on data from different fish groups. The results showed a significant correlation between GTA data and production estimates, with important correlations for tilapia (R²=0.69) and trout (R²=0.55). When the previous year's production was added to the models as a predictor variable, the models' R² values for tilapia and trout increased to 0.89 and 0.80, respectively. The validation of these two models for 2022 and 2023 indicated good performance, with an excellent fit between the model's predictions and Epagri’s estimates (R² above 0.95). However, the model developed for trout tended to underestimate production. It is concluded that the models produced are effective in predicting the commercial production of tilapia and, to a lesser extent, trout in Santa Catarina.
Metrics
Publication Facts
Reviewer profiles N/A
Author statements
- Academic society
- Epagri - Revista Agropecuária Catarinense
- Publisher
- Empresa de Pesquisa Agropecuária e Extensão Rural de Santa Catarina - Epagri
References
CAMPBELL, T.; DIXON, K. W.; DODS, K.; FEARNS, P.; HANDCOCK, R. Machine Learning Regression Model for Predicting Honey Harvests. Agriculture, v.10, n.4, p.1-17, 2020. DOI: https://doi.org/10.3390/agriculture10040118
EPAGRI. Observatório Agro Catarinense. Epagri, Centro de Socioeconomia e Planejamento Agrícola. Disponível em: https://www.observatorioagro.sc.gov.br/areas-tematicas/producao-agropecuaria/paineis/ Acesso em: 24 jul. 2024.
HERRERA, L.J.; POMARES, H.; ROJAS, I.; GUILLÉN, A.; PRIETO, A.; Valenzuela, O. Recursive prediction for long term time series forecasting using advanced models. Neurocomputing, v.70, n.16–18, p. 2870-2880, 2007. DOI: https://doi.org/10.1016/j.neucom.2006.04.015.
OTWINOWSKA-MINDUR, A.; GUMUŁKA, M.; KANIA-GIERDZIEWICZ, J. Mathematical Models for Egg Production in Broiler Breeder Hens. Annals of Animal Science, v.16, n.4., p. 1185–1198, 2016. Disponível em: https://sciendo.com/article/10.1515/aoas-2016-0037. Acesso em: 04 abr. 2025.
RANAPURWALA, S.I.; CAVANAUGH, J.E.; YOUNG, T.; WU, H.; PEEK-ASA, C.; RAMIREZ, M.R. Public health application of predictive modeling: an example from farm vehicle crashes. Injury Epidemiology, v.6, 31. p. 1-11, 2019. DOI: https://doi.org/10.1186/s40621-019-0208-9
SADENOVA M.A.; BEISEKENOV N.A.; RAKHYMBERDINA M.Y.; VARBANOV P.S.; KLEMEŠ J.J. Mathematical Modelling in Crop Production to Predict Crop Yields. Chemical Engineering Transactions, v. 88, 1225-1230, 2021. DOI: https://doi.org/10.1515/aoas-2016-0037
SILVA, B. C. da; DELLA GIUSTINA, E. G.; MARCHIORI, N. da C.; MASSAGO, H.; SILVA, F. M. Desempenho produtivo da piscicultura catarinense. Agropecuária Catarinense, v.30, n.1, p.15–18, 2017. Disponível em: https://publicacoes.epagri.sc.gov.br/rac/article/view/176. Acesso em: 4 abr. 2025.
SOUZA, R. V. de .; SILVA, B. C. da; NOVAES, A. L. T. A aquicultura de Santa Catarina em números. Documentos, (354), 2022. Disponível em: https://publicacoes.epagri.sc.gov.br/DOC/article/view/1532. Acesso em: 04 abr. 2025.
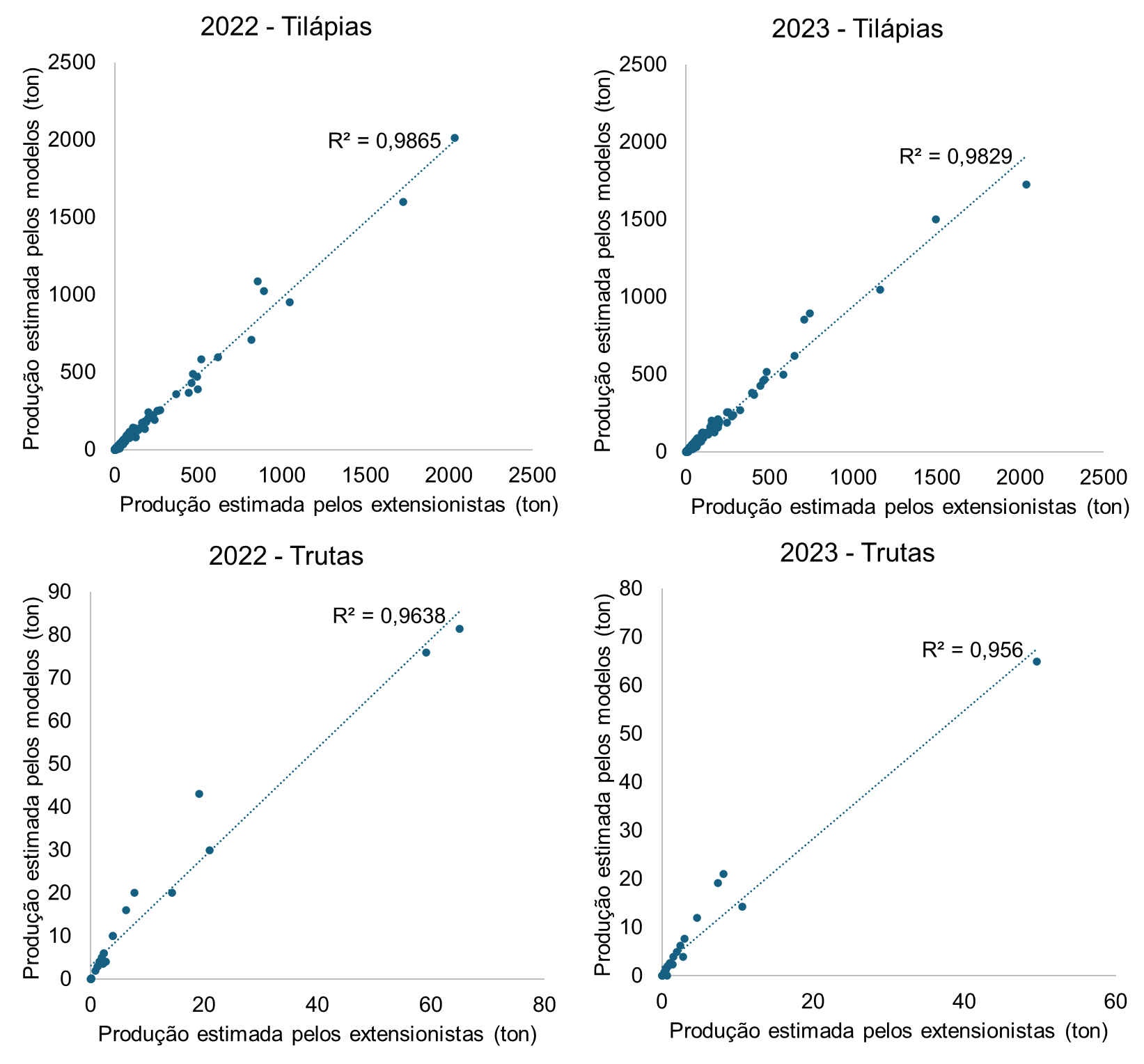
Downloads
Published
How to Cite
Issue
Section
License
Copyright (c) 2025 Robson Ventura de Souza, André Luis Tortato Novaes

This work is licensed under a Creative Commons Attribution 4.0 International License.