Use of soil spectral reflectance to estimate texture for agricultural insurance purposes
Keywords:
Agricultural Zoning for Climatic Risk, soil textural classes, soil available water, machine learningAbstract
The Agricultural Zoning for Climatic Risk is an important tool for risk management that uses soil parameters to determine the climatic risk associated with cultivating specific crops. This study aimed to evaluate the performance of soil reflectance spectroscopy combined with a machine learning algorithm in predicting soil clay and sand contents for agricultural insurance purposes. A confusion matrix was used to classify samples measured in the laboratory and predicted by the Cubist algorithm. The results showed that Cubist demonstrated good predictive performance with R² = 0.83, RMSE = 7.29%, and RPIQ = 3.70 for clay, and R² = 0.73, RMSE = 10.56%, and RPIQ = 2.64 for sand. In the confusion matrix, most samples were correctly classified into the soil types defined by IN 02/2008, with an overall accuracy of 80%. Considering IN, there was a clear difference in the soil available water values. The study demonstrates the potential of spectroscopy combined with machine learning algorithms in predicting soil attributes as a complementary technique in agricultural risk management.
Metrics
Publication Facts
Reviewer profiles N/A
Author statements
- Academic society
- Epagri - Revista Agropecuária Catarinense
- Publisher
- Empresa de Pesquisa Agropecuária e Extensão Rural de Santa Catarina - Epagri
References
AGÊNCIA NACIONAL DE ÁGUAS E SANEAMENTO BÁSICO – COORDENAÇÃO DE ESTUDOS SETORIAIS (Brasília). Capacidade de água disponível – CAD ou AWC dos solos do Brasil. Brasília: ANA, 2021. 1 mapa. Escala 1:250.000. Disponível em: https://metadados.snirh.gov.br/geonetwork/srv/api/records/28fe4baa-66f3-4f6b-b0d2-890abf5910c4. Acesso em: 19 agosto 2023.
AHMADI, A.; EMAMI, M.; DACCACHE, A.; HE, L. Soil properties prediction for precision agriculture using visible and near-infrared spectroscopy: A systematic review and meta-analysis. Agronomy, v.11, 2021. DOI: https://doi.org/10.3390/agronomy11030433.
ARAÚJO FILHO, J.C.; BARROS, A.H.C; GALVÃO, P.V.M.;TEIXEIRA, W.G.; LIMA, E.P.; VICTORIA, D.C.; ANDRADE JUNIOR, A.S.; XAVIER, J.P.S; LUMBRERAS, J.F.; COELHO, M.R.; MANSILLA BACA, J.F.; MONTEIRO, J.E.B.A.; OLIVEIRA, F.C.S.F.; SILVA FILHO, A.D.; BARROS, J.P.F.G. Avaliação, predição e mapeamento de água disponível em solos do Brasil. Rio de Janeiro: Embrapa Solos. 2022. (Boletim de pesquisa e desenvolvimento/Embrapa Solos, ISSN 1678-0892; 282). Disponível em: https://www.embrapa.br/busca-de-publicacoes/-/publicacao/1143345/avaliacao-predicao-e-mapeamento-de-agua-disponivel-em-solos-do-brasil. Acesso em: 19 agosto 2023.
BAUMANN, P.; HELFENSTEIN, A.; GUBLER, A.; KELLER, A.; MEULI, R. G.; WÄCHTER, D.; LEE, J., VISCARRA ROSSEL, R.; SIX, J. Developing the Swiss mid-infrared soil spectral library for local estimation and monitoring. Soil, v.7, 2021. DOI: https://doi.org/10.5194/soil-7-525-2021.
BENEDETTI, F.; van EGMOND, F. Global Soil Spectroscopy Assessment. Spectral soil data: needs and capacities. Rome: FAO, 2021. DOI: https://doi.org/10.4060/cb6265en.
BORTOLINI, D.; ALBUQUERQUE, J.A. Estimation of the retention and availability of water in soil of the state of Santa Catarina. Revista Brasileira de Ciência do Solo, v.42, e0170250, 2018. DOI: https://doi.org/10.1590/18069657rbcs20170250.
BRASIL. Instrução Normativa no 2 de 09 de outubro de 2008. Brasília: Ministério da Agricultura, Pecuária e Abastecimento, [2008]. Disponível em: https://www.gov.br/agricultura/pt-br/assuntos/riscos-seguro/programa-nacional-de-zoneamento-agricola-de-risco-climatico/documentos/INn2de09.10.2008.pdf/view. Acesso em: 10 abril 2016.
BRASIL. Instrução Normativa no 1 de 21 de junho de 2022. Brasília: Ministério da Agricultura, Pecuária e Abastecimento, [2022]. Disponível em: https://www.gov.br/agricultura/pt-br/assuntos/riscos-seguro/programa-nacional-de-zoneamento-agricola-de-risco-climatico/documentos/InstruoNormativan1de21dejunhode2022site.pdf/view. Acesso em: 10 jul 2022.
CEZAR, E.; NANNI, M.R.; GUERRERO, C.; SILVA JUNIOR, C.A.; CRUCIOL, L.G.T.; CHICATI, M.L.; SILVA, G.F.C. Organic matter and sand estimates by spectroradiometry: strategies for the development of models with applicability at a local scale. Geoderma, v.340, 2019. DOI: https://doi.org/10.1016/j.geoderma.2019.01.021.
COSTA, A.; ALBUQUERQUE, J.A.; COSTA, A.; PÉRTILE, P.; SILVA, F.R. Water retention and availability in soils of the state of Santa Catarina-Brasil: Effect of textural classes, soil classes and lithology. Revista Brasileira de Ciência do Solo, v.37, 2013. DOI: https://doi.org/10.1590/S0100-06832013000600010.
DE CARVALHO, A. L.; SANTOS, D. V.; MARENGO, J. A., COUTINHO; S. M. V.; MAIA, S. M. F. Impacts of extreme climate events on Brazilian agricultural production. Sustentabilidade em Debate, v.11, 2020. DOI: https://doi.org/10.18472/SustDeb.v11n3.2020.33814.
DEMATTÊ, J. A. M.; FONGARO, C. T.; RIZZO, R.; SAFANELLI, J. L. Geospatial Soil Sensing System (GEOS3): A powerful data mining procedure to retrieve soil spectral reflectance from satellite images. Remote Sensing of Environment, v.212, 2018. DOI: https://doi.org/10.1016/j.rse.2018.04.047.
DEMATTÊ, J.A.M; DOTTO, A.D.; PAIVA, A.F.S., SATO, M.V.; DALMOLIN, R.S.D.; ARAÚJO, M.S.B.; SILVA, E.B.; NANNI, M.R.; TEN CATEN, A.; NORONHA, N.C.; LACERDA, M.P.C.; ARAÙJO FILHO. J.C.; RIZZO, R. BELLINASO, H.; FRANCELINO, M.R.; SCHAEFEN, C.E.G.R.; VICENTE, L.E.; SANTOS, U.J.; SAMPAIO, E.V.S.B.; MENEZES, R.S.C.; SOUZA, J.J.L.L; ABRAHÃO, W.A.P.; COELHO, R.M.; GREGO, C.R.; LANI, J.L.; FERNANDES, A.R.; GONÇALVES, D.A.M.; SILVA, S.H.G.; MENEZES, M.D.; CURI, N.; COUTO, E.G.; ANJOS, L.H.C.; CEDDIA, M.B.; PINHEIRO, E.F.M.; GRUNWALD, S.; VASQUES, G.M.; MARQUES JÚNIOR, J.; SILVA, A.J.; BARRETO, M.C.V.; NÓBREGA, G.N.; SILVA, M.Z.; SOUZA, S.F.; VALLADARES, G.S.; VIANA, J.H.M.; TERRA, F.S.; HORÁK-TERRA, I.; FIORIO, P.R.; SILVA, R.C.; FRADE JÚNIOR, E.F.; LIMA, R.H.C.; ALBA, J.M.F.; SOUZA JUNIOR, V.S.; SANTOS BREFIN, M.L.M.; RUIVO, M.L.P.; FERREIRA, T.O.; BRAIT, M.A.; CAETANO, N.R.; BRINGHENTI, I.; MENDES, W.S.; SAFANELLI, J.L.; GUIMARAES, C.C.B.; POPPIEL, R.R.; BARROS E SOUZA, A.; QUESADA, C.A.; COUTO, H.T.Z. The Brazilian Soil Spectral Library (BSSL): A general view, application and challenges. Geoderma, v.354, 2019a. DOI: https://doi.org/10.1016/j.geoderma.2019.05.043.
DEMATTÊ, J.A.M.; DOTTO, A.C.; BEDIN, L.G.; SAYÃO, V.M.; BARROS E SOUZA, A. Soil analytical quality control by traditional and spectroscopy techniques: Constructing the future of a hybrid laboratory for low environmental impact. Geoderma, v.337, 2019b. DOI: https://doi.org/10.1016/j.geoderma.2018.09.010.
DEMATTÊ, J.A.M; PAIVA, A.F.S., POPPIEL, R.R.; ROSIN, N.A.; RUIZ, L.F.C.; MELLO, F.A.O.; MINASNY, B.; GRUNWALD, S.; GE, Y.; BEM DOR, E.; GHOLIZADEH, A.; GOMEZ, C.; CHABRILLAT, S.; FRANCOS, N.; AYOUBI, S.; FIANTIS, D.; BINEY, J.K.M.; WANG, C.; BELAL, A.; NAIMI, S.; HAFSHEJANI, N.A.; BELLINASO, H.; MOURA-BUENO, J.M.; SILVERO, N.E.Q. The Brazilian Soil Spectral Service (BraSpecS): A User‐Friendly System for Global Soil Spectra Communication. Remote Sensing, v.14, 2022. DOI: https://doi.org/10.3390/rs14030740.
DOTTO, A. C.; DALMOLIN, R.S.D.; ten CATEN, A.; MOURA-BUENO, J.M. Potential of spectroradiometry to classify soil clay content. Revista Brasileira de Ciência do Solo, Viçosa, v.40, 2016. DOI: https://doi.org/10.1590/18069657rbcs20151105.
FRANCESCHINI, M. H.D.; DEMATTÊ, J.A.M.; SATO, M.V.; VICENTE, L.E.; GREGO, C.R. et al. Abordagens semiquantitativa e quantitativa na avaliação da textura do solo por espectroscopia de reflectância bidirecional no VIS-NIR-SWIR. Pesquisa Agropecuária Brasileira, Brasília, v.48, 2013. DOI: http://dx.doi.org/10.1590/S0100-204X2013001200006.
HOLMES, G.; HALL, M.; PRANK, E. Generating rule sets from model trees. In: AUSTRALIAN JOINT CONFERENCE ON ARTIFICIAL INTELLIGENCE, 12., 1999, Sydney, Proceedings[…] Berlin, 1999.
HOSSEINI, E.; ZAREI, M.; MOOSAVI, A.A.; GHASEMI-FASAEI, R.; BAGHERNEJAD, M.; MOZAFFARI, H. Feasibility of Vis-NIR spectroscopy approach to predict soil biological attributes in arid land soils. PLos ONE, v.19, e0311122, 2024. DOI: https://doi.org/10.1371/journal.pone.0311122.
JACONI, A.; VOS, C.; DON, A. Soil texture - Near infrared spectroscopy as an easy and precise method to estimate soil texture. Geoderma, v.337, 2019. DOI: https://doi.org/10.1016/j.geoderma.2018.10.038.
LACERDA, M.; DEMATTÊ, J.A.M; SATO, M.V.; FONGARO, C.T.; GALLO, B.C.; SOUZA, A.B. Tropical texture determination by proximal sensing using a regional spectral library and its relationship with soil classification. Remote Sensing, v. 8, 2016. DOI: https://doi.org/10.3390/rs8090701.
MELSER, D.; LE, T.; RUTHBAH, U. Climate change and its impact on home insurance uptake in Australia. Ecological Economics, v.222, 2024. DOI: https://doi.org/10.1016/j.ecolecon.2024.108195.
MENDES, W.S.; DEMATTÊ, J. A. M.; ROSIN, N. A.; TERRA, F. S.; POPPIEL, R. R.; URBINA-SALAZAR, D. F.; BOECHAT, C. L.; SILVA, E. B.; CURI, N., SILVA, S. H. G.; Uemeson, J.S.; Valladares, G.S. The Brazilian soil Mid-infrared Spectral Library: The Power of the Fundamental Range. Geoderma, v.415, 2022. https://doi.org/10.1016/j.geoderma.2022.115776.
MENDES, W.S.; MEDEIROS NETO, L. G.; DEMATTÊ, J. A. M.; GALLO, B. C.; RIZZO, R.; SAFANELLI, J. L.; FONGARO, C. T. Is it possible to map subsurface soil attributes by satellite spectral transfer models? Geoderma, v.343, 2019. DOI: https://doi.org/10.1016/j.geoderma.2019.01.025.
OECD-FAO. Agricultural Outlook 2021-2030. OECD Publishing, Paris, 2021. DOI: https://doi.org/10.1787/19991142.
PAIVA, A. F. S.; POPPIEL, R. R.; ROSIN, N. A.; GRESCHUK, L. T.; ROSAS, J. T. F.; DEMATTÊ, J. A. M. The Brazilian Program of soil analysis via spectroscopy (ProBASE): Combining spectroscopy and wet laboratories to understand new technologies. Geoderma, v.421, 2022. DOI: https://doi.org/10.1016/j.geoderma.2022.115905.
PICCINI, C.; METZGER, K.; DEBAENE, G.; STENBERG, B.; GÖTZINGER, S.; BORŮVKA, L.; SANDÉN, T.; BRAGAZZA, L.; LIEBISCH, F. In-field soil spectroscopy in Vis–NIR range for fast and reliable soil analysis: A review. In European Journal of Soil Science, v.75, 2024. DOI: https://doi.org/10.1111/ejss.13481.
R CORE TEAM. R: A language and environment for statistical computing. R version 4.1.1. Viena, Áustria: R Foundation for Statistical Computing, 2021. Disponível em: <https://www.R-project.org/>. Acesso em: 10 abril 2023.
ROMERO, D. J.; BEN-DOR, E.; DEMATTÊ, J.A.M.; BARROS e SOUZA, A.; VICENTE, L.E.; TAVARES, T.R.; MARTELLO, M.; STRABELI, T.F.; BARROS, P.P.S.; FIORIO, P.R.; GALLO, B.C.; SATO, M.V.; EITELWEIN, M.T. Internal soil standard method for the Brazilian soil spectral library: Performance and proximate analysis. Geoderma, v.312, 2018. DOI: https://doi.org/10.1016/j.geoderma.2017.09.014
SHEPHERD, K.D.; FERGUSON, R.; HOOVER, D.; VAN EGMOND, F.; SANDERMAN, J.; GE, Y. A global soil spectral calibration library and estimation service. Soil Security, v.7, 2022. DOI: https://doi.org/10.1016/j.soisec.2022.100061.
SILVA, E. B.; GIASSON, É.; DOTTO, A. C.; ten CATEN, A.; DEMATTÊ, J. A. M., BACIC, I. L. Z., VEIGA, M. A regional legacy soil dataset for prediction of sand and clay content with VIS-NIR-SWIR, in southern Brazil. Revista Brasileira de Ciência Do Solo, v.43, 2019. DOI: https://doi.org/10.1590/18069657rbcs20180174.
SORIANO-DISLA, J. M.; JANIK, L. J.; VISCARRA-ROSSEL, R. A.; MACDONALD, L. M.; MCLAUGHLIN, M. J. The performance of visible, near-, and mid-infrared reflectance spectroscopy for prediction of soil physical, chemical, and biological properties. Applied Spectroscopy Reviews, v.49, 2014. DOI: https://doi.org/10.1080/05704928.2013.811081.
STEVENS, A.; TÓTH M.N.G; MONATARELLA, L.; VAN WESEMAEL, B. Prediction of Soil Organic Carbon at the European scale by visible and near infrared reflectance spectroscopy. PLosOne, v.19, 2013. DOI: http://doi: 10.1371/journal.pone.0066409.
SUMMERAUER, L.; BAUMANN, P.; RAMIREZ-LOPEZ, L.; BARTHEL, M.; BAUTERS, M.; BUKOMBE, B.; REICHENBACH, M.; BOECKX, P.; KEARSLEY, E.; VAN OOST, K.; VANLAUWE, B.; CHIRAGAGA, D.; HERI-KAZI, A. B.; MOONEN, P.; SILA, A.; SHEPHERD, K.; BAZIRAKE MUJINYA, B.; VAN RANST, E.; BAERT, G.; SIX, J. The central African soil spectral library: A new soil infrared repository and a geographical prediction analysis. Soil, v.7, 2021. DOI: https://doi.org/10.5194/soil-7-693-2021
TEIXEIRA, W.G.; VICTORIA, D.C.; BARROS, A.H.C.; LUMBRERAS, J.F.; ARAÚJO FILHO, J.C.; SILVA, F.A.M.; LIMA, E.P.; BUENO FILHO, J.S.S.; MONTEIRO, J.E.B.A. Predição de água disponível no solo em função da granulometria para uso nas análises de risco no zoneamento agrícola de risco climático. Rio de Janeiro: Embrapa Solos, 2021. (Boletim de pesquisa e desenvolvimento/Embrapa Solos, 272).
THOMAS, C. L.; HERNANDEZ-ALLICA, J.; DUNHAM, S. J.; MCGRATH, S. P.; HAEFELE, S. M. A comparison of soil texture measurements using mid-infrared spectroscopy (MIRS) and laser diffraction analysis (LDA) in diverse soils. Scientific Reports, v.11, 2021. DOI: https://doi.org/10.1038/s41598-020-79618-y
VASQUES, G.M.; DEMATTÊ, J.A.M.; VISCARRA ROSSEL, R.A.; RAMIREZ-LÓPEZ, L.; TERRA, F.S. Soil classification using visible/near-infrared diffuse reflectance spectra from multiple depths. Geoderma, v.223-225, 2014. DOI: https://doi.org/10.1016/j.geoderma.2014.01.019.
VEIGA, M.; SANTOS, O. V.; HAMMES, L. A.; PANDOLFO, C. Distribuição espacial dos teores de argila, silte e areia na camada superficial do solo em Santa Catarina. Revista Agropecuária Catarinense, Florianópolis, SC, v.25, n.1, p. 63-68, 2012.
VISCARRA ROSSEL, R. A.; WEBSTER, R. Predicting soil properties from the Australian soil visible-near infrared spectroscopic database. European Journal of Soil Science, v. 63, 2012. DOI: https://doi.org/10.1111/j.1365-2389.2012.01495.x.
ZENG, R.; ROSSITER, D.G.; YANG, F.; LI, D-C.; ZHAO, Y-G.; ZHANG, G-L. How accurately can soil classes be allocated based on spectrally predicted physio-chemical properties. Geoderma, v.303, 2017. DOI: https://doi.org/10.1016/j.geoderma.2017.05.011.
ZHANG, M.; SHI, W.; XU, Z. Systematic comparison of machine-learning models in classification and interpolation of soil particle size fractions using diferent transformed data. Hydrology and Earth System Sciences, v.24, 2020. DOI: https://doi.org/10.5194/hess-24-2505-2020.
ZHANG, X., XUE, J., XIAO, Y., SHI, Z., CHEN, S. Towards Optimal Variable Selection Methods for Soil Property Prediction Using a Regional Soil Vis-NIR Spectral Library. Remote Sensing, v.15, 2023. DOI: https://doi.org/10.3390/rs15020465.
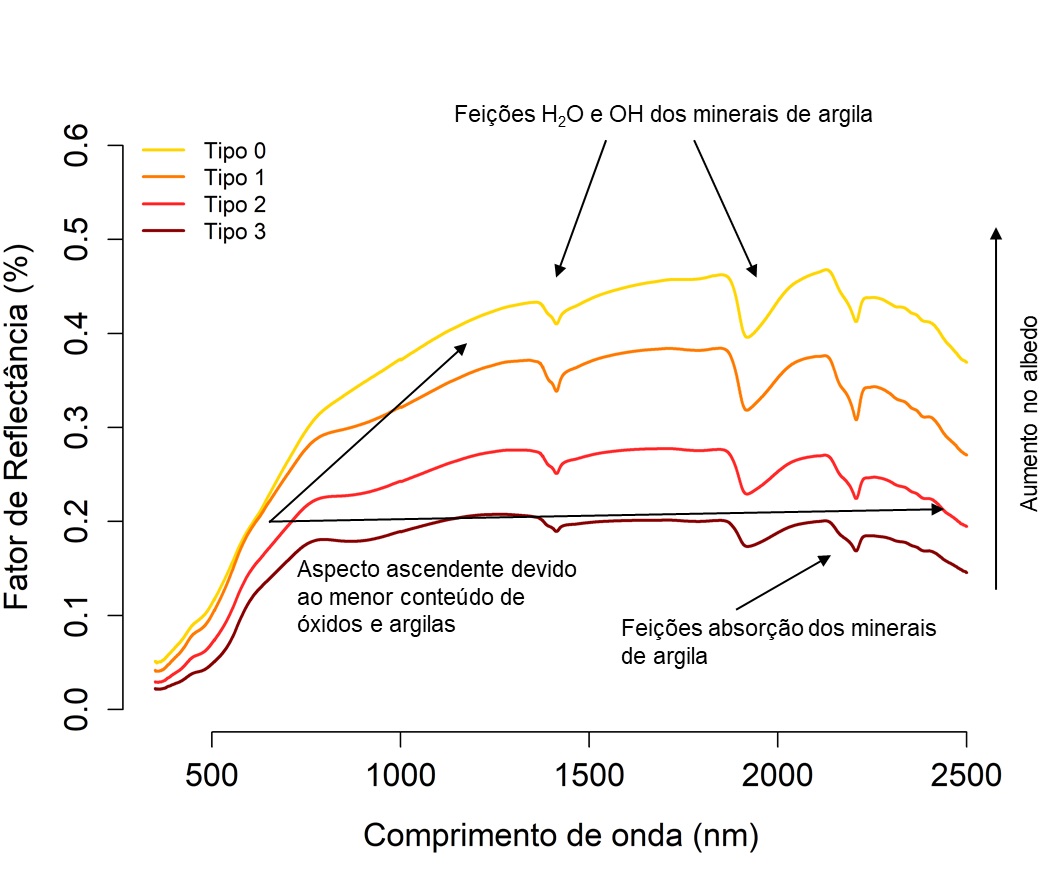
Downloads
Published
How to Cite
Issue
Section
License
Copyright (c) 2024 Elisângela Benedet da Silva, Cristina Pandolfo, Gabriel Berenhauser Leite, Élvio Giasson

This work is licensed under a Creative Commons Attribution 4.0 International License.